Course Description
This course examines the fundamentals of detection and estimation for signal processing, communications, and control. Topics covered include: vector spaces of random variables; Bayesian and Neyman-Pearson hypothesis testing; Bayesian and nonrandom parameter estimation; minimum-variance unbiased estimators and the …
This course examines the fundamentals of detection and estimation for signal processing, communications, and control. Topics covered include: vector spaces of random variables; Bayesian and Neyman-Pearson hypothesis testing; Bayesian and nonrandom parameter estimation; minimum-variance unbiased estimators and the Cramer-Rao bounds; representations for stochastic processes, shaping and whitening filters, and Karhunen-Loeve expansions; and detection and estimation from waveform observations. Advanced topics include: linear prediction and spectral estimation, and Wiener and Kalman filters.
Course Info
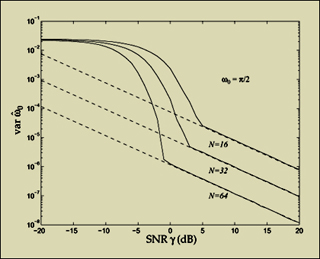
Example of threshold phenomenon in nonlinear estimation. (Image courtesy of Alan Willsky and Gregory Wornell.)